Comparing Soil Sampling Strategies - Machine Learning vs Grid
Traditional grid soil sampling and machine learning-driven soil sample designs represent two distinct approaches to discovering soil sample locations and density, each with unique advantages and limitations. Grid sampling involves collecting soil samples at regular intervals across a field,typically at a single depth, and analysing a limited number of analytes. The sampling density for grid sampling is usually around 1 core per 2 hectares.This approach provides a flat, 2D snapshot of soil conditions, which may not capture the 3 dimensional complexity of factors like compaction, nutrient distribution, sodicity, acidity, and salinity.
In contrast, OptiSoil’s machine learning-driven soil sampling designs, leverage proximal sensing tools like dual electromagnetic (EM) and gamma radio metrics to account for soil variability at a much finer resolution. These tools are used to contrast soil properties such as clay content, salt, and moisture across the field, creating a detailed baseline map of soil variability.
Machine learning algorithms then use this data along with landscape attributes to design optimised sampling plans, ensuring samples are taken from areas that best represent the field's variability. This approach requires significantly fewer cores generally around 25-30 cores for a 1,000 hectare area and analyses a larger number of analytes across multiple depths,extending into the root zone, to create a comprehensive 3D map of soil characteristics. There is an investment trade-off between the two methods: grid sampling requires more cores but analyses fewer depths and analytes, while machine learning-driven sampling uses far fewer cores but provides more detailed data across multiple depths and analytes often for a similar cost.
A critical feature of this approach is that the predictions made by machine learning are always validated with maps of uncertainty. These maps quantify the confidence level of the predictions, helping farmers understand the reliability and value of the data. For instance, areas with low uncertainty indicate high confidence in the predicted soil properties, while areas with high uncertainty may require additional sampling or further investigation. This transparency ensures that decision-making is based on robust,scientifically validated information. While grid sampling remains a simpler option and has a place in some circumstances, machine learning-driven solutions with proximal sensing represent the future of precision agriculture
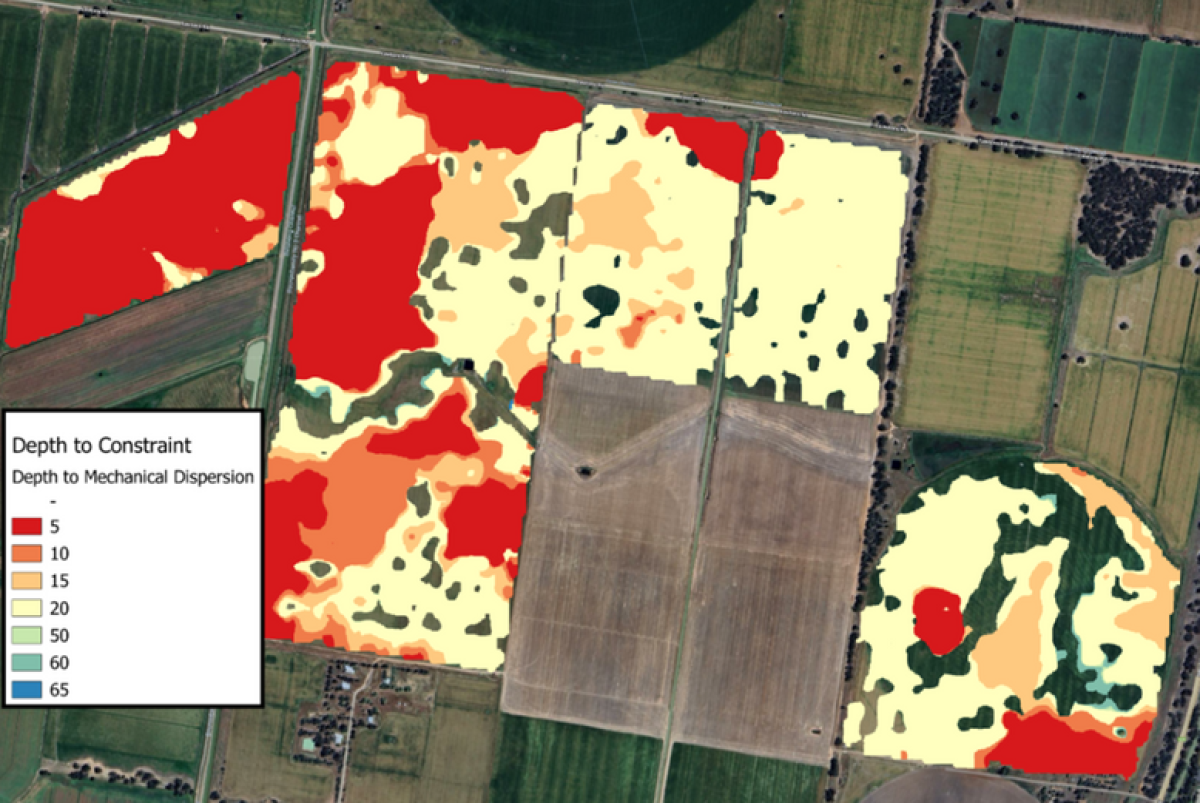