Better Soil Decisions with EM, Gamma and Machine Learning
Proximal sensing methods, particularly the integration of electromagnetic (EM) induction and gamma radiometric surveys, have become invaluable tools in modern agriculture for mapping soil variability and informing fertiliser and soil amelioration strategies.
These technologies, when combined with machine learning algorithms, enable the development of precise soil sampling designs that account for spatial variability, leading to more effective soil management practices.
EM induction measures the soil's apparent electrical conductivity (ECa), which is influenced by factors such as soil moisture, salinity, and clay content. Higher ECa readings often indicate increased moisture levels, higher clay content, or elevated salinity.
Gamma radiometric surveys, on the other hand, detect natural gamma radiation emitted by isotopes like potassium-40, uranium, and thorium in the soil. These measurements provide insights into soil mineral composition and texture, as different soil types emit varying levels of gamma radiation.
The integration of EM and gamma radiometric data allows for a more comprehensive understanding of soil properties.
For instance, combining these datasets can effectively delineate different soil types and identify subsoil constraints, such as acidity or compaction layers, which are critical for determining appropriate amelioration techniques.
Machine learning algorithms can process the extensive datasets generated by these proximal sensing methods to create detailed soil property maps. By analysing patterns and relationships within the data, machine learning models can predict soil constraints and moisture levels across a farm. This predictive capability supports the development of targeted soil sampling strategies, ensuring that sampling efforts are both efficient and representative of the field's variability.
In summary, the combined use of EM induction and gamma radiometric surveys, enhanced by machine learning, offers a robust framework for understanding soil variability. This integrated approach enables the development of precise soil sampling designs and informed amelioration strategies, ultimately leading to improved soil health and agricultural productivity.
Key advantages of integrating EM with gamma radiometrics over EM alone
- Improved Soil Texture Differentiation – EM measures soil electrical conductivity, which can be influenced by moisture, salinity, and clay content, making it difficult to distinguish between these factors. Gamma radiometrics provides additional data on soil mineral composition and texture, allowing for more accurate soil classification. For instance, in the diverse soils of New South Wales, combining EM and gamma radiometric data has enabled more accurate soil classification, distinguishing between sandy and clayey soils, which is crucial for soil core site selection.
- Better Identification of Subsoil Constraints to around 40cm – EM alone may not effectively detect issues like compacted layers, acidity, or sodicity in deeper soil horizons. Combining EM with gamma radiometrics improves the ability to map these contrasting soil types leading to identification of constraints and guide targeted amelioration strategies.
- More Accurate Soil Variability Mapping – Gamma radiometrics provides spatial information about soil parent material, which EM alone cannot measure. This additional layer of information improves soil mapping and helps refine precision agriculture practices.
- Enhanced Machine Learning Predictions – Machine learning models benefit from multi-sensor datasets. By integrating gamma data with EM, predictive models can better distinguish soil property variations, leading to more reliable soil sampling designs and management decisions.
References
- Using ground-based EM and radiometrics to map soil amelioration, July 2020 GRDC Groundcover. Using-ground-based-em-and-radiometrics-to-map-soil-amelioration
- Using EM and gamma maps to map soil types and help locate sub soil constraints for management. GRDC Update paper, Oliver, Wong, Mata, Holmes, February 2019. Using-em-and-gamma-maps-to-map-soil-types-and-help-locate-subsoil-constraints-for-management
- Examples of using machine learning for mapping soil constraints and soil moisture to support improved decision making. GRDC Update paper, February 2021, Bishop, Fillipi. Examples-of-using-machine-learning-for-mapping-soil-constraints-and-soil-moisture-to-support-improved-decision-making
- 3D maps take soils management to new levels. Grain Automate and Digital agronomy, May-June 2024, Wang 3d-maps-take-soils-management-to-new-levels
- Mapping soil properties and their impact on yield - combining Dual EM, gamma radiometrics, elevation and soil colour to select sampling sites to predict soil properties and investigate their impact on yield across the paddock. GRDC Update paper February 2020. Jones, Hulme, Malone, Fillipi, McBratney. mapping-soil-properties-and-their-impact-on-yield-combining-dual-em -gamma-radiometrics%2C-elevation-and-soil-colour-to-select-sampling-sites-to-predict-soil-properties-and-investigate-their-impact-on-yield-across-the-paddock
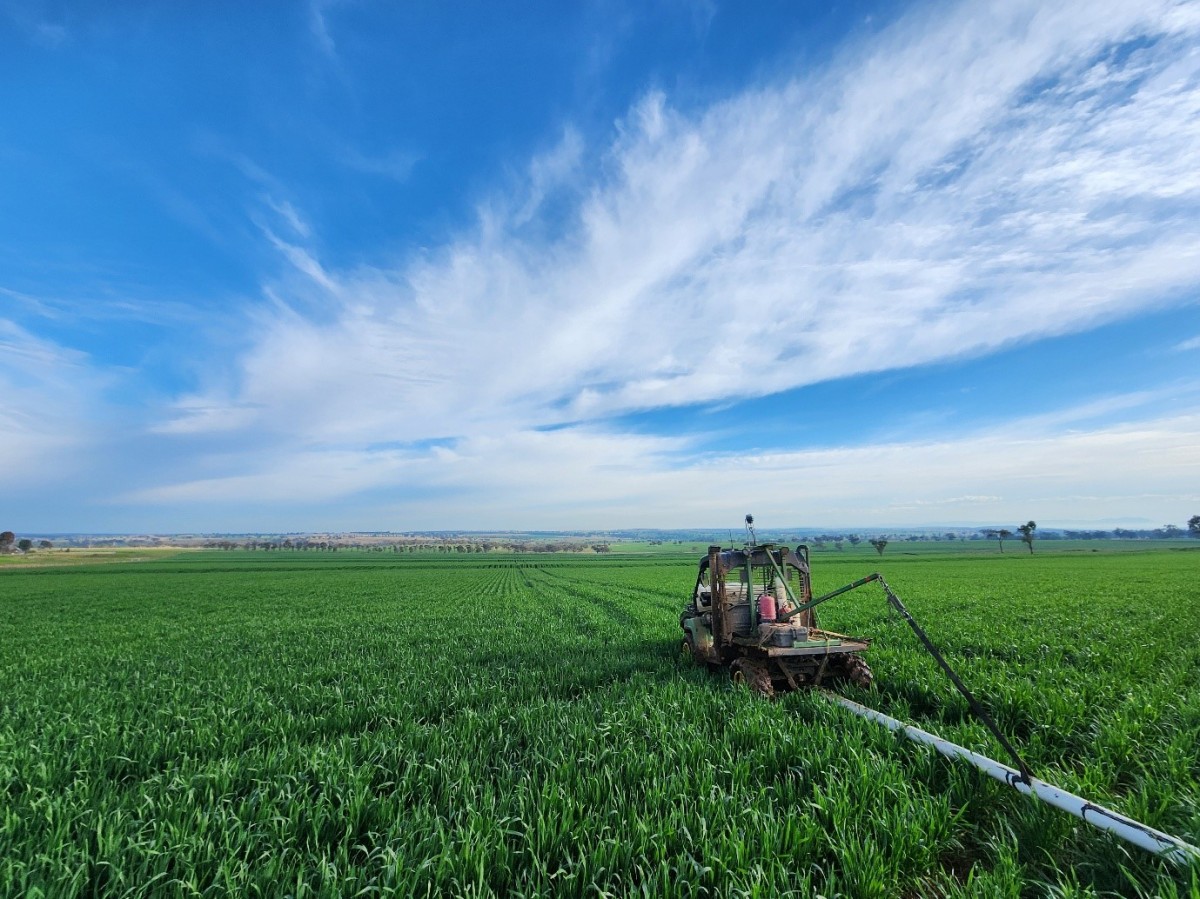
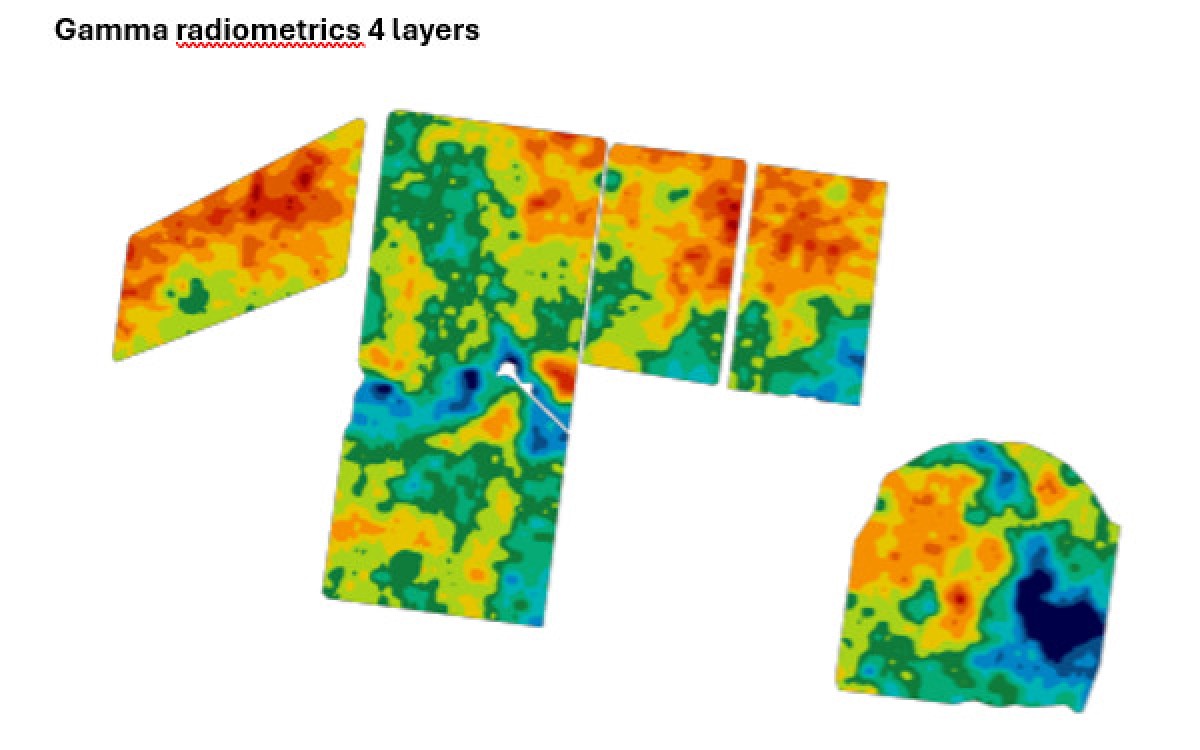
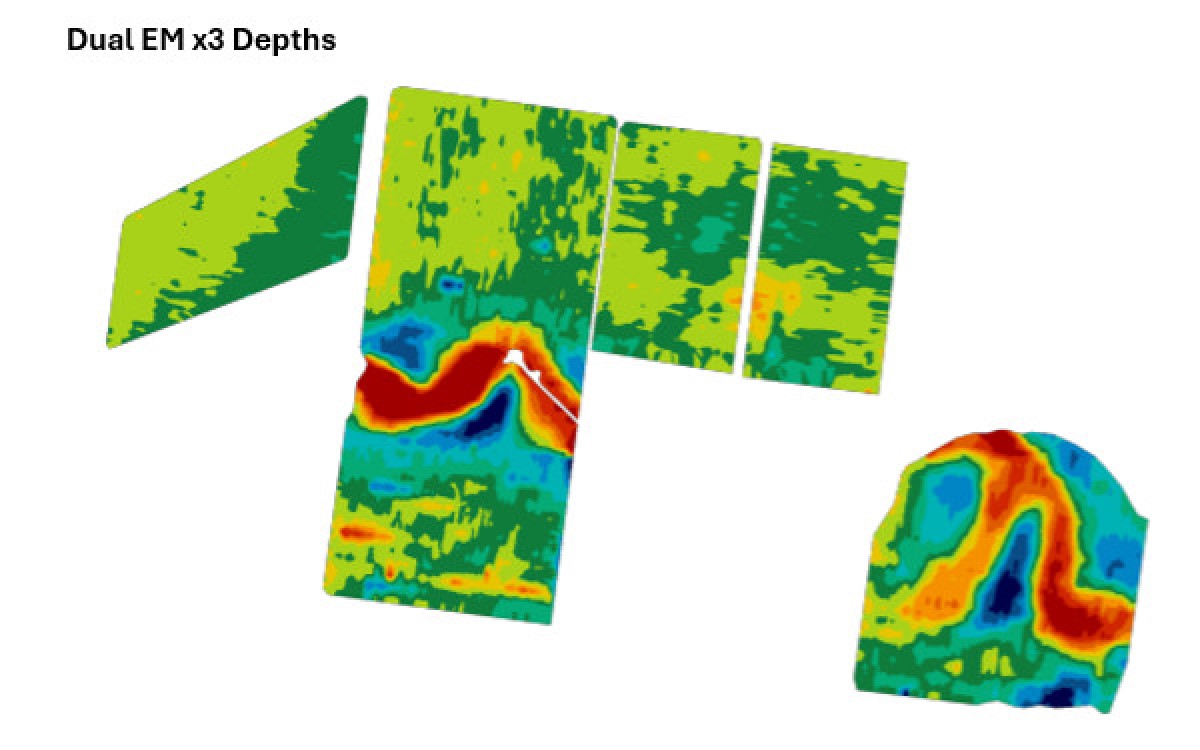
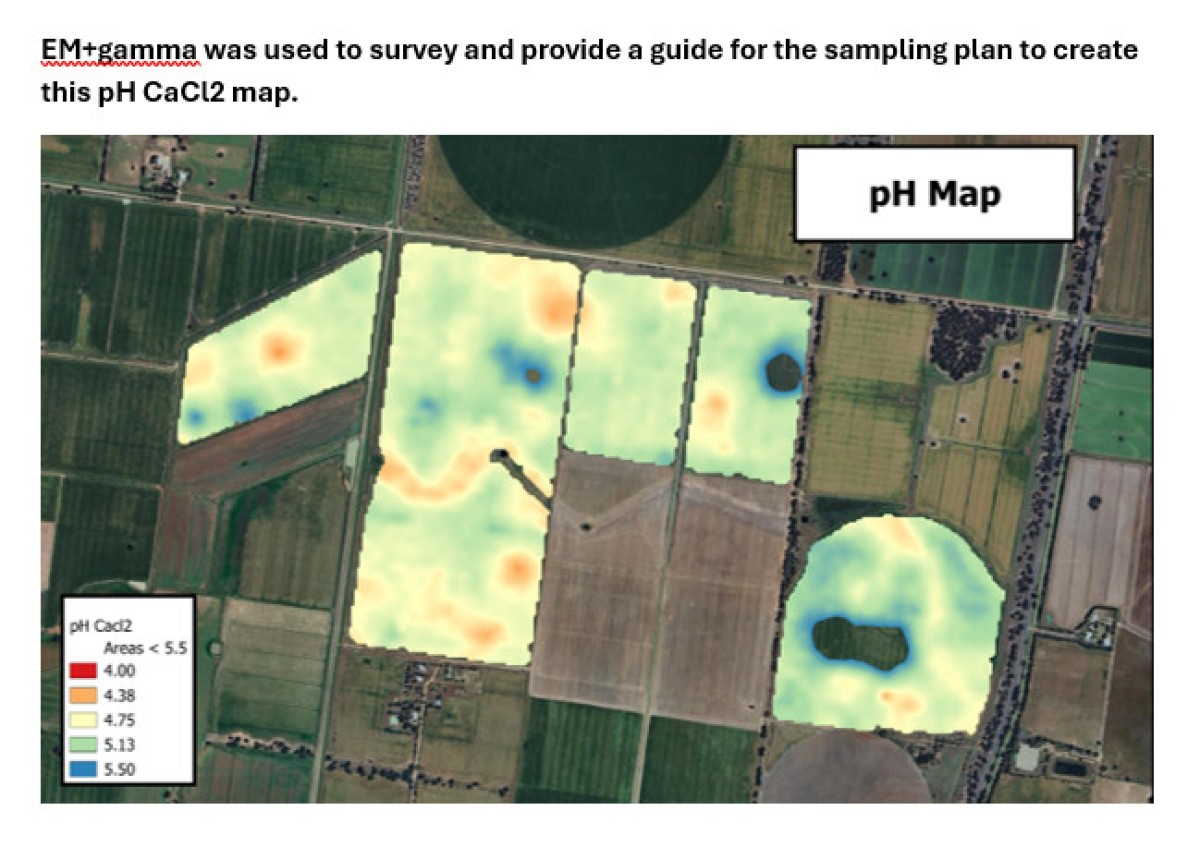
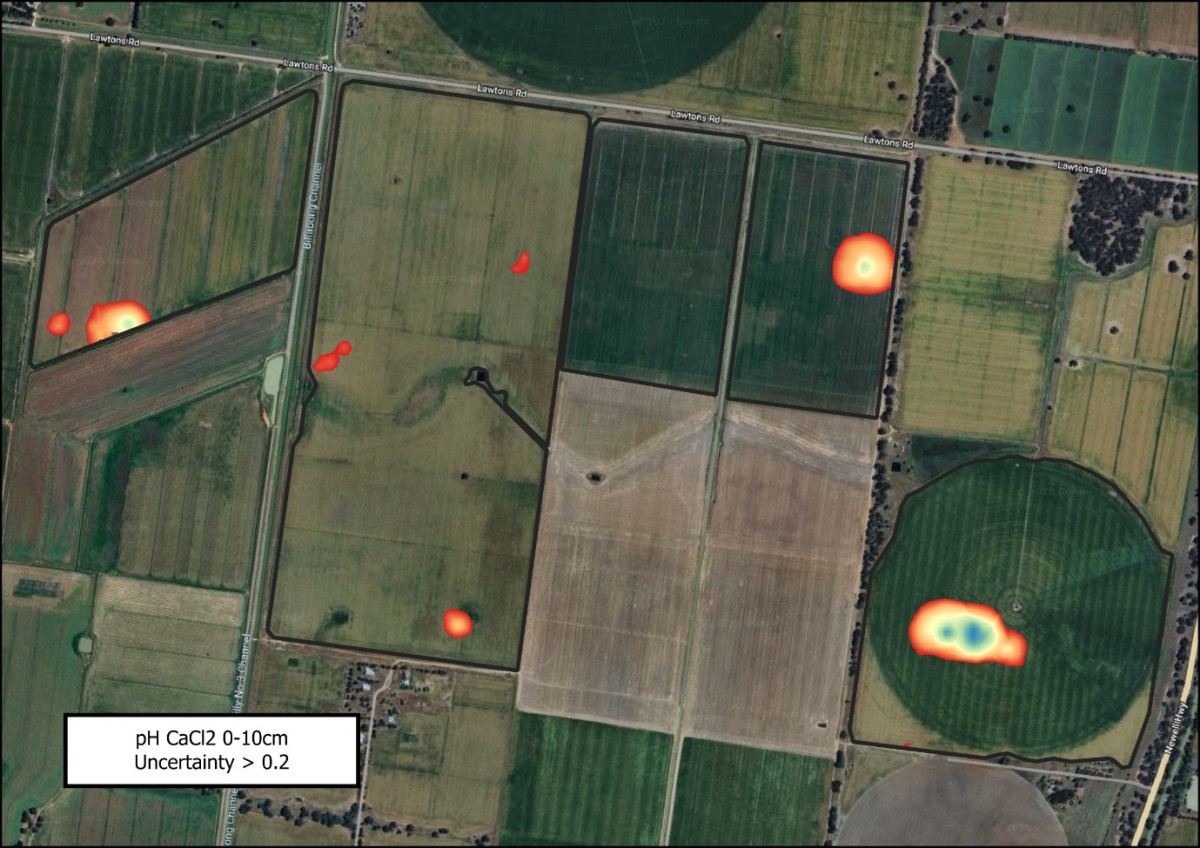